The Future of Manufacturing: How AI is Transforming the Industry
The manufacturing sector has just begun realizing the potential of artificial intelligence in manufacturing to transform legacy processes and operations. The technology is advancing further, and adoption is accelerating, and AI in manufacturing is expected to create over $20.8 billion in value for the industry globally by 2028.
The landscape of the 21st century demands better quality, higher efficiency, lower costs and sustainability, and AI and manufacturing will be key to gaining the competitive edge needed in such a world. Manufacturing AI is the next big productivity driver the industry must embrace.
Key AI Use Cases Driving Transformation in Manufacturing
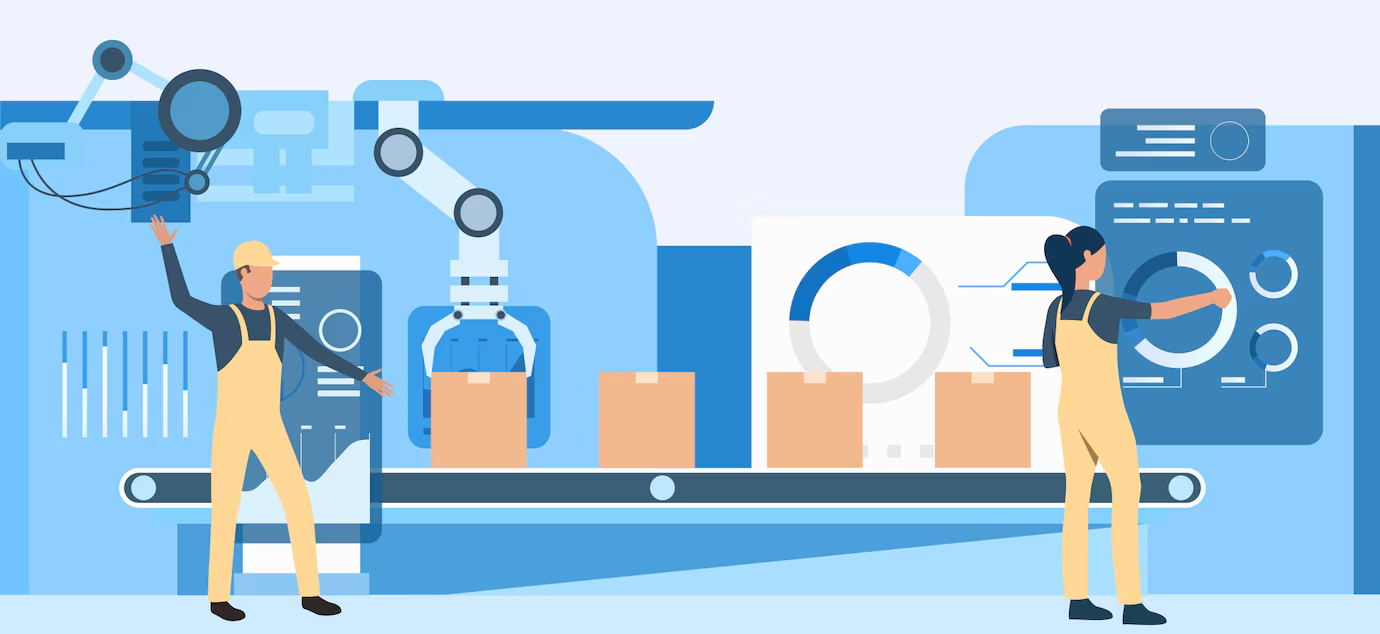
Manufacturing stands at a pivotal juncture where adopting AI for manufacturing, through manufacturing software development services is set to make the difference between industry leaders and laggards. Artificial intelligence in manufacturing industry has graduated from hype to real results across operations. Scaling AI deployments in production will separate the best from the rest.
1. Generative Design
Generative design is an AI methodology that mimics nature’s evolutionary approach to come up with new design ideas. It uses algorithms to rapidly explore permutations against specified constraints to create thousands of design options non-intuitively. Engineers then select the best design for prototyping.
The technology provides completely new perspectives on solving design challenges, leading to lighter, stronger and more sustainable products. For instance, manufacturing artificial intelligence helped redesign an aircraft partition to reduce weight by 45% while retaining strength. AI in production unlocks better design.
2. Predictive Quality
Quality issues can proliferate into expensive field failures in manufacturing. AI vision inspection systems can now reliably detect defects in products and components early. Combining visual AI monitoring data with predictive analytics provides alerts on potential quality problems even before they occur.
Instead of inspecting quality at the end of the production line, manufacturers can embed predictive quality measures throughout the process, enabling proactive control. AI drives smarter quality control.
3. Predictive Maintenance
Equipment failures are unanticipated, and they cause expensive production delays and maintenance costs. AI predictive maintenance uses telemetry data to find early signs of wear and tear and predict the remaining useful life of a component. It alerts you in time on assets that require preventive maintenance.
AI in the manufacturing industry delivers over 50% reduction in unplanned downtime, allowing better production planning and asset utilization. Early failure prediction is invaluable.
4. Demand Forecasting
Aligning production with demand requires good visibility of upcoming requirements. AI manufacturing utilizes machine learning to process sales data, external indicators, and sensor signals to deliver a 15-20% improvement in demand forecast accuracy compared to traditional statistical methods.
Better demand prediction provides manufacturers with data-driven inputs for production planning and inventory optimization, driving growth and profitability. AI makes demand sensing smarter.
5. Production Scheduling
Efficient scheduling of machines, workers, buffers, and material flow is key to high utilization in capital-intensive manufacturing. Mathematical programming techniques used in AI software can dynamically allocate constrained resources to optimize production schedules on the fly.
Manufacturing AI handles hundreds of simultaneous operational constraints to enhance output, quality, and flow. Improved scheduling translates directly to higher margins.
Key Takeaways on Adopting AI in Manufacturing Industry
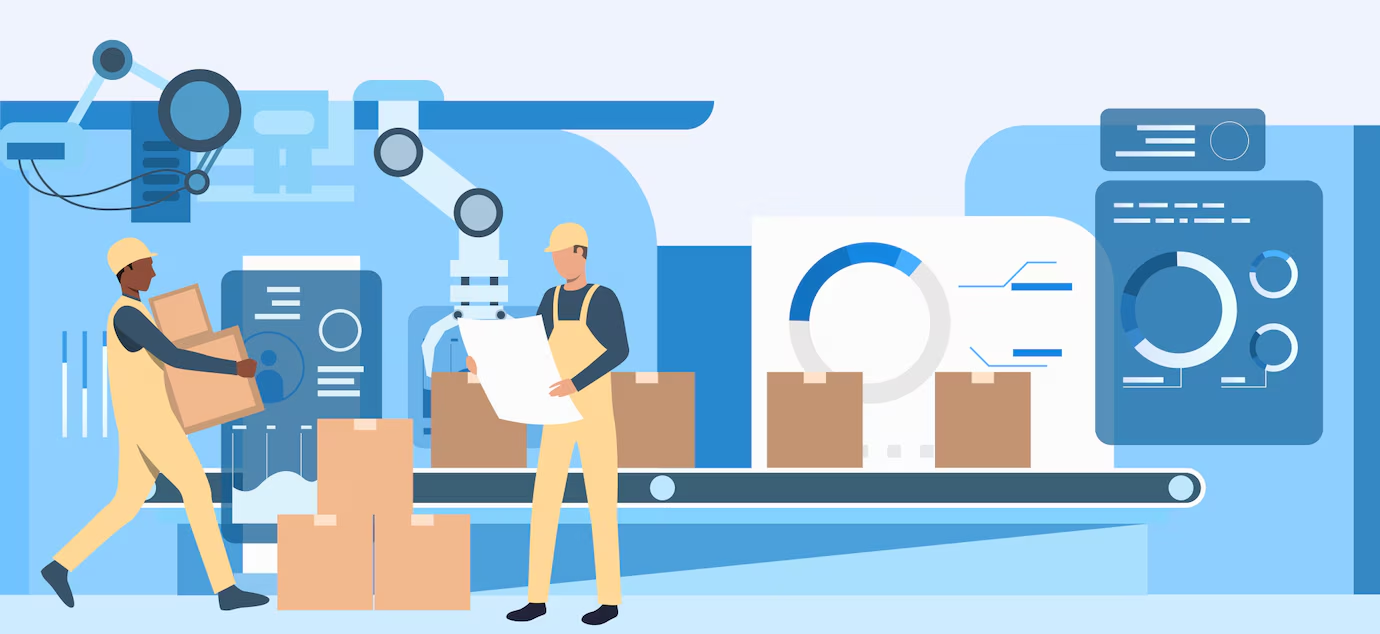
The impact of AI adoption across the value chain is poised to propel manufacturing to the edge of a revolution. Leading manufacturers have already demonstrated the substantial financial and operational benefits of early AI implementations. To fully realize AI’s potential, businesses need to strategically scale AI in the manufacturing industry by focusing on four key aspects:
Process-centric Perspective
Focus on the operational impact rather than the theoretical appeal of AI. Choose use cases that directly address pain points preventing production, quality or planning from happening.
Data Readiness
Feed AI algorithms clean and connected data sets assembled across manufacturing processes. Better data requires better instrumentation and connectivity.
AI Talent Development
Kick-off internal training programs and hire lateral AI talent who understands manufacturing operations as well as data science. Build an AI CoE.
Change Management
Help stakeholders understand what AI can and can’t do and what the timelines are. Sandboxes are used to test new AI solutions before enterprise-wide adoption. Make results measured transparently.
The right strategy, plus sustained investment in AI, will separate manufacturing leaders from the pack. AI will drive the future of manufacturing.